1 2 3 4 5 6 7 8 9 10 11 12 13 14 15 16 17 18 19 20 21 22 23 24 25 26 27 28 29 30 31 32 33 34 35 36 37 38 39 40 41 42 43 44 45 46 47 48 49 50 51 52 53 54 55 56 57 58 59 60 61 62 63 64 65 66 67 68 69 70 71 72 73 74 75 76 77 78 79 80 81 82 83 84 85 86 87 88 89 90 91 92 93 94 95 96 97 98 99 100 101 102 103 104 105 106 107 108 109 110 111 112 113 114 115 116 117 118 119 120 121 122 123 124 125 126 127 128 129 130 131 132 133 134 135 136 137 138 139 140 141 142 143 144 145 146 147 148 149 150 151 152 153 154 155 156 157 158 159 160 161 162 163 164 165 166 167 168 169 170 171 172 173 174 175 176 177 178 179 180 181 182 183 184 185 186 187 188 189 190 191 192 193 194 195 196 197 198 199 200 201 202 203 204 205 206 207 208 209 210 211 212 213 214 215 216 217 218 219 220 221 222 223 224 225 226 227 228 229 230 231 232 233 234 235 236 237 238 239 240 241 242 243 244 245 246 247 248 249 250 251 252 253 254 255 256 257 258 259 260 261 262 263 264 265 266 267 268 269 270 271 272 273 274 275 276 277 278 279 280 281 282 283 284 285 286 287 288 289 290 291 292 293 294 295 296 297 298 299 300 301 302 303 304 305 306 307 308 309 310 311 312 313 314 315 316 317 318 319 320 321 322 323 324 325 326 327 328 329 330 331 332 333 334 335 336 337 338 339 340 341 342 343 344 345 346 347 348 349 350 351 352 353 354 355 356 357 358 359 360 361 362 363 364 365 366 367 368 369 370 371 372 373 374 375 376 377 378 379 380 381 382 383 384 385 386 387 388 389 390 391 392 393 394 395 396 397 398 399 400 401 402 403 404 405 406 407 408 409 410 411 412 413 414 415 416 417 418 419 420 421 422 423 424 425 426 427 428 429 430 431 432 433 434 435 436 437 438 439 440 441 442 443 444 445 446 447 448 449 450 451 452 453 454 455 456 457 458 459 460 461 462 463 464 465 466 467 468 469 470 471 472 473 474 475 476 477 478 479 480 481 482 483 484 485 486 487 488 489 490 491 492 493 494 495 496 497 498 499 500 501 502 503 504 505 506 507 508 509 510 511 512 513 514 515 516 517 518 519 520 521 522 523 524 525 526 527 528 529 530 531 532 533 534 535 536 537 538 539 540 541 542 543 544 545 546 547 548 549 550 551 552 553 554 555 556 557 558 559 560 561 562 563 564 565 566 567 568 569 570 571 572 573 574 575 576 577 578 579 580 581 582 583 584 585 586 587 588 589 590 591 592 593 594 595 596 597 598 599 600 601 602 603 604 605 606 607 608 609 610 611 612 613 614 615 616 617 618 619 620 621 622 623 624 625 626 627 628 629 630 631 632 633 634 635 636 637 638 639 640 641 642 643 644 645 646 647 648 649 650 651 652 653 654 655 656 657 658 659 660 661 662 663 664 665 666 667 668 669 670 671 672 673 674 675 676 677 678 679 680 681 682 683 684 685 686 687 688 689 690 691 692 693 694 695 696 697 698 699 700 701 702 703 704 705 706 707 708 709 710 711 712 713 714 715 716 717 718 719 720 721 722 723 724 725 726 727 728 729 730 731 732 733 734 735 736 737 738 739 740 741 742 743 744 745 746 747 748 749 750 751 752 753 754 755 756 757 758 759 760 761 762 763 764 765 766 767 768 769 770 771 772 773 774 775 776 777 778 779 780 781 782 783 784 785 786 787 788 789 790 791 792 793 794 795 796 797 798 799 800 801 802 803 804 805 806 807 808 809 810 811 812 813 814 815 816 817 818 819 820 821 822 823 824 825 826 827 828 829 830 831 832 833 834 835 836 837 838 839 840 841 842 843 844 845 846 847 848 849 850 851 852 853 854 855 856 857
#![allow( unused_parens, clippy::excessive_precision, clippy::missing_safety_doc, clippy::not_unsafe_ptr_arg_deref, clippy::should_implement_trait, clippy::too_many_arguments, clippy::unused_unit, )] //! # Surface Matching //! //! Note about the License and Patents //! ----------------------------------- //! //! The following patents have been issued for methods embodied in this //! software: "Recognition and pose determination of 3D objects in 3D scenes //! using geometric point pair descriptors and the generalized Hough //! Transform", Bertram Heinrich Drost, Markus Ulrich, EP Patent 2385483 //! (Nov. 21, 2012), assignee: MVTec Software GmbH, 81675 Muenchen //! (Germany); "Recognition and pose determination of 3D objects in 3D //! scenes", Bertram Heinrich Drost, Markus Ulrich, US Patent 8830229 (Sept. //! 9, 2014), assignee: MVTec Software GmbH, 81675 Muenchen (Germany). //! Further patents are pending. For further details, contact MVTec Software //! GmbH (info@mvtec.com). //! //! Note that restrictions imposed by these patents (and possibly others) //! exist independently of and may be in conflict with the freedoms granted //! in this license, which refers to copyright of the program, not patents //! for any methods that it implements. Both copyright and patent law must //! be obeyed to legally use and redistribute this program and it is not the //! purpose of this license to induce you to infringe any patents or other //! property right claims or to contest validity of any such claims. If you //! redistribute or use the program, then this license merely protects you //! from committing copyright infringement. It does not protect you from //! committing patent infringement. So, before you do anything with this //! program, make sure that you have permission to do so not merely in terms //! of copyright, but also in terms of patent law. //! //! Please note that this license is not to be understood as a guarantee //! either. If you use the program according to this license, but in //! conflict with patent law, it does not mean that the licensor will refund //! you for any losses that you incur if you are sued for your patent //! infringement. //! //! //! Introduction to Surface Matching //! -------------------------------- //! //! Cameras and similar devices with the capability of sensation of 3D structure are becoming more //! common. Thus, using depth and intensity information for matching 3D objects (or parts) are of //! crucial importance for computer vision. Applications range from industrial control to guiding //! everyday actions for visually impaired people. The task in recognition and pose estimation in range //! images aims to identify and localize a queried 3D free-form object by matching it to the acquired //! database. //! //! From an industrial perspective, enabling robots to automatically locate and pick up randomly placed //! and oriented objects from a bin is an important challenge in factory automation, replacing tedious //! and heavy manual labor. A system should be able to recognize and locate objects with a predefined //! shape and estimate the position with the precision necessary for a gripping robot to pick it up. //! This is where vision guided robotics takes the stage. Similar tools are also capable of guiding //! robots (and even people) through unstructured environments, leading to automated navigation. These //! properties make 3D matching from point clouds a ubiquitous necessity. Within this context, I will //! now describe the OpenCV implementation of a 3D object recognition and pose estimation algorithm //! using 3D features. //! //! Surface Matching Algorithm Through 3D Features //! ---------------------------------------------- //! //! The state of the algorithms in order to achieve the task 3D matching is heavily based on //! [drost2010](https://docs.opencv.org/4.3.0/d0/de3/citelist.html#CITEREF_drost2010), which is one of the first and main practical methods presented in this area. The //! approach is composed of extracting 3D feature points randomly from depth images or generic point //! clouds, indexing them and later in runtime querying them efficiently. Only the 3D structure is //! considered, and a trivial hash table is used for feature queries. //! //! While being fully aware that utilization of the nice CAD model structure in order to achieve a smart //! point sampling, I will be leaving that aside now in order to respect the generalizability of the //! methods (Typically for such algorithms training on a CAD model is not needed, and a point cloud //! would be sufficient). Below is the outline of the entire algorithm: //! //! 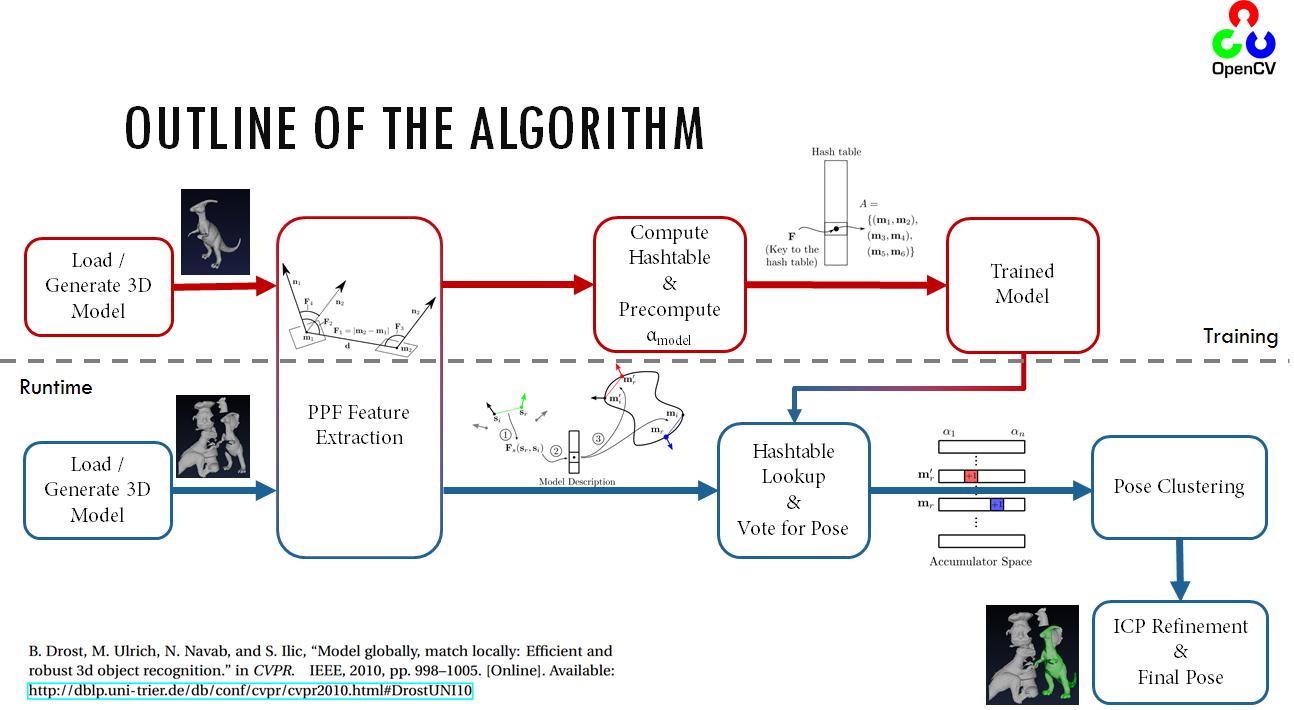 //! //! As explained, the algorithm relies on the extraction and indexing of point pair features, which are //! defined as follows: //! //!  //! //! where  and  are feature two selected points on the model (or scene), //!  is the difference vector,  and  are the normals at  and //! . During the training stage, this vector is quantized, indexed. In the test stage, same //! features are extracted from the scene and compared to the database. With a few tricks like //! separation of the rotational components, the pose estimation part can also be made efficient (check //! the reference for more details). A Hough-like voting and clustering is employed to estimate the //! object pose. To cluster the poses, the raw pose hypotheses are sorted in decreasing order of the //! number of votes. From the highest vote, a new cluster is created. If the next pose hypothesis is //! close to one of the existing clusters, the hypothesis is added to the cluster and the cluster center //! is updated as the average of the pose hypotheses within the cluster. If the next hypothesis is not //! close to any of the clusters, it creates a new cluster. The proximity testing is done with fixed //! thresholds in translation and rotation. Distance computation and averaging for translation are //! performed in the 3D Euclidean space, while those for rotation are performed using quaternion //! representation. After clustering, the clusters are sorted in decreasing order of the total number of //! votes which determines confidence of the estimated poses. //! //! This pose is further refined using  in order to obtain the final pose. //! //! PPF presented above depends largely on robust computation of angles between 3D vectors. Even though //! not reported in the paper, the naive way of doing this ( //! remains numerically unstable. A better way to do this is then use inverse tangents, like: //! //!  //! //! Rough Computation of Object Pose Given PPF //! ------------------------------------------ //! //! Let me summarize the following notation: //! //! * :  point of the model ( accordingly) //! * : Normal of the  point of the model ( accordingly) //! * :  point of the scene ( accordingly) //! * : Normal of the  point of the scene ( accordingly) //! * : The transformation required to translate  to the origin and rotate //! its normal  onto the -axis. //! * : Rotational component of . //! * : Translational component of . //! * :  point of the model transformed by . ( //! accordingly). //! * : Axis angle representation of rotation . //! * : The angular component of the axis angle representation //! . //! //! The transformation in a point pair feature is computed by first finding the transformation //!  from the first point, and applying the same transformation to the second one. //! Transforming each point, together with the normal, to the ground plane leaves us with an angle to //! find out, during a comparison with a new point pair. //! //! We could now simply start writing //! //!  //! //! where //! //!  //! //! Note that this is nothing but a stacked transformation. The translational component //!  reads //! //!  //! //! and the rotational being //! //!  //! //! in axis angle format. Note that bold refers to the vector form. After this transformation, the //! feature vectors of the model are registered onto the ground plane X and the angle with respect to //!  is called . Similarly, for the scene, it is called . //! //! ### Hough-like Voting Scheme //! //! As shown in the outline, PPF (point pair features) are extracted from the model, quantized, stored //! in the hashtable and indexed, during the training stage. During the runtime however, the similar //! operation is perfomed on the input scene with the exception that this time a similarity lookup over //! the hashtable is performed, instead of an insertion. This lookup also allows us to compute a //! transformation to the ground plane for the scene pairs. After this point, computing the rotational //! component of the pose reduces to computation of the difference . This //! component carries the cue about the object pose. A Hough-like voting scheme is performed over the //! local model coordinate vector and . The highest poses achieved for every scene point lets us //! recover the object pose. //! //! ### Source Code for PPF Matching //! //! ~~~{cpp} //! // pc is the loaded point cloud of the model //! // (Nx6) and pcTest is a loaded point cloud of //! // the scene (Mx6) //! ppf_match_3d::PPF3DDetector detector(0.03, 0.05); //! detector.trainModel(pc); //! vector<Pose3DPtr> results; //! detector.match(pcTest, results, 1.0/10.0, 0.05); //! cout << "Poses: " << endl; //! // print the poses //! for (size_t i=0; i<results.size(); i++) //! { //! Pose3DPtr pose = results[i]; //! cout << "Pose Result " << i << endl; //! pose->printPose(); //! } //! ~~~ //! //! Pose Registration via ICP //! ------------------------- //! //! The matching process terminates with the attainment of the pose. However, due to the multiple //! matching points, erroneous hypothesis, pose averaging and etc. such pose is very open to noise and //! many times is far from being perfect. Although the visual results obtained in that stage are //! pleasing, the quantitative evaluation shows  degrees variation (error), which is an acceptable //! level of matching. Many times, the requirement might be set well beyond this margin and it is //! desired to refine the computed pose. //! //! Furthermore, in typical RGBD scenes and point clouds, 3D structure can capture only less than half //! of the model due to the visibility in the scene. Therefore, a robust pose refinement algorithm, //! which can register occluded and partially visible shapes quickly and correctly is not an unrealistic //! wish. //! //! At this point, a trivial option would be to use the well known iterative closest point algorithm . //! However, utilization of the basic ICP leads to slow convergence, bad registration, outlier //! sensitivity and failure to register partial shapes. Thus, it is definitely not suited to the //! problem. For this reason, many variants have been proposed . Different variants contribute to //! different stages of the pose estimation process. //! //! ICP is composed of  stages and the improvements I propose for each stage is summarized below. //! //! ### Sampling //! //! To improve convergence speed and computation time, it is common to use less points than the model //! actually has. However, sampling the correct points to register is an issue in itself. The naive way //! would be to sample uniformly and hope to get a reasonable subset. More smarter ways try to identify //! the critical points, which are found to highly contribute to the registration process. Gelfand et. //! al. exploit the covariance matrix in order to constrain the eigenspace, so that a set of points //! which affect both translation and rotation are used. This is a clever way of subsampling, which I //! will optionally be using in the implementation. //! //! ### Correspondence Search //! //! As the name implies, this step is actually the assignment of the points in the data and the model in //! a closest point fashion. Correct assignments will lead to a correct pose, where wrong assignments //! strongly degrade the result. In general, KD-trees are used in the search of nearest neighbors, to //! increase the speed. However this is not an optimality guarantee and many times causes wrong points //! to be matched. Luckily the assignments are corrected over iterations. //! //! To overcome some of the limitations, Picky ICP [pickyicp](https://docs.opencv.org/4.3.0/d0/de3/citelist.html#CITEREF_pickyicp) and BC-ICP (ICP using bi-unique //! correspondences) are two well-known methods. Picky ICP first finds the correspondences in the //! old-fashioned way and then among the resulting corresponding pairs, if more than one scene point //!  is assigned to the same model point , it selects  that corresponds to the minimum //! distance. BC-ICP on the other hand, allows multiple correspondences first and then resolves the //! assignments by establishing bi-unique correspondences. It also defines a novel no-correspondence //! outlier, which intrinsically eases the process of identifying outliers. //! //! For reference, both methods are used. Because P-ICP is a bit faster, with not-so-significant //! performance drawback, it will be the method of choice in refinment of correspondences. //! //! ### Weighting of Pairs //! //! In my implementation, I currently do not use a weighting scheme. But the common approaches involve //! *normal compatibility* () or assigning lower weights to point pairs with //! greater distances (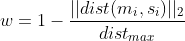). //! //! ### Rejection of Pairs //! //! The rejections are done using a dynamic thresholding based on a robust estimate of the standard //! deviation. In other words, in each iteration, I find the MAD estimate of the Std. Dev. I denote this //! as . I reject the pairs with distances . Here  is the threshold of //! rejection and by default set to . The weighting is applied prior to Picky refinement, explained //! in the previous stage. //! //! ### Error Metric //! //! As described in , a linearization of point to plane as in [koklimlow](https://docs.opencv.org/4.3.0/d0/de3/citelist.html#CITEREF_koklimlow) error metric is used. This //! both speeds up the registration process and improves convergence. //! //! ### Minimization //! //! Even though many non-linear optimizers (such as Levenberg Mardquardt) are proposed, due to the //! linearization in the previous step, pose estimation reduces to solving a linear system of equations. //! This is what I do exactly using cv::solve with DECOMP_SVD option. //! //! ### ICP Algorithm //! //! Having described the steps above, here I summarize the layout of the ICP algorithm. //! //! #### Efficient ICP Through Point Cloud Pyramids //! //! While the up-to-now-proposed variants deal well with some outliers and bad initializations, they //! require significant number of iterations. Yet, multi-resolution scheme can help reducing the number //! of iterations by allowing the registration to start from a coarse level and propagate to the lower //! and finer levels. Such approach both improves the performances and enhances the runtime. //! //! The search is done through multiple levels, in a hierarchical fashion. The registration starts with //! a very coarse set of samples of the model. Iteratively, the points are densified and sought. After //! each iteration the previously estimated pose is used as an initial pose and refined with the ICP. //! //! #### Visual Results //! //! ##### Results on Synthetic Data //! //! In all of the results, the pose is initiated by PPF and the rest is left as: //!  //! //! ### Source Code for Pose Refinement Using ICP //! //! ~~~{cpp} //! ICP icp(200, 0.001f, 2.5f, 8); //! // Using the previously declared pc and pcTest //! // This will perform registration for every pose //! // contained in results //! icp.registerModelToScene(pc, pcTest, results); //! //! // results now contain the refined poses //! ~~~ //! //! Results //! ------- //! //! This section is dedicated to the results of surface matching (point-pair-feature matching and a //! following ICP refinement): //! //! 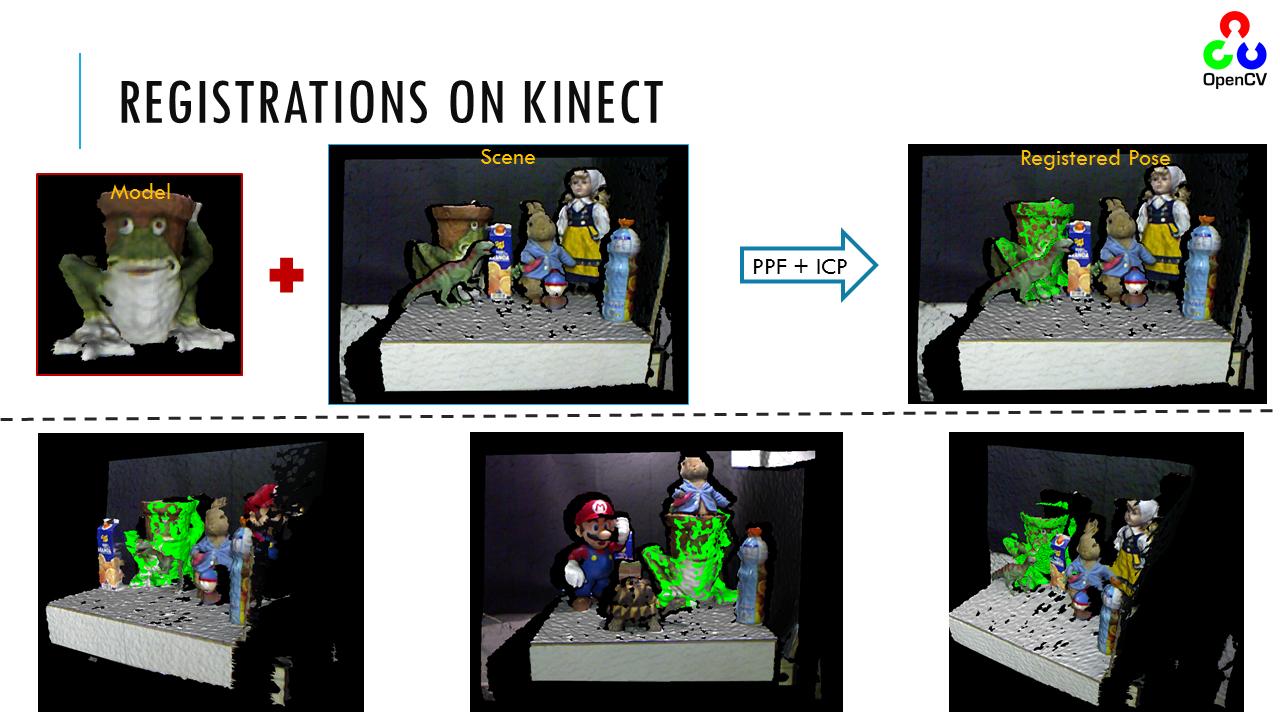 //! //! Matches of different models for Mian dataset is presented below: //! //! 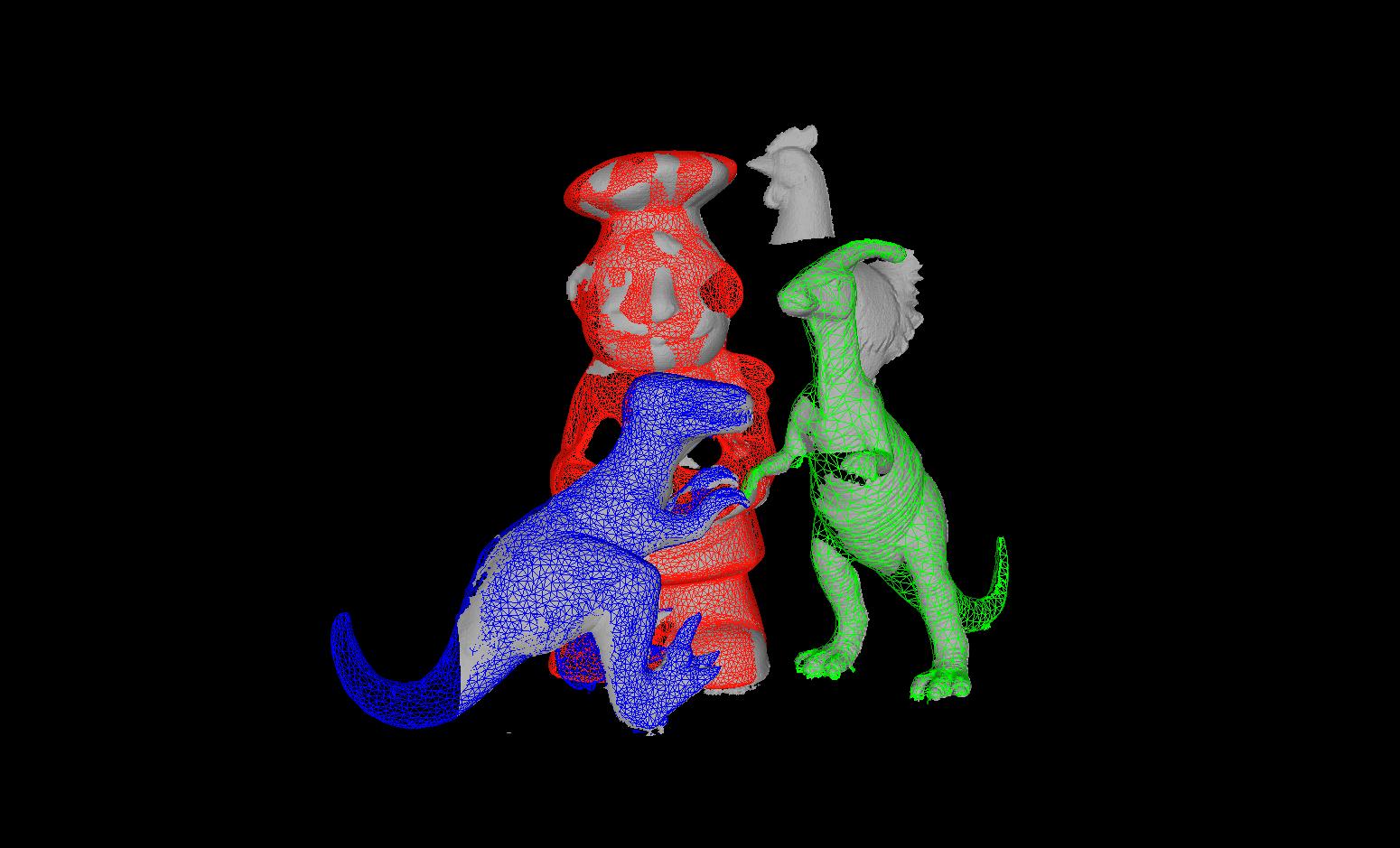 //! //! You might checkout the video on [youTube here](http://www.youtube.com/watch?v=uFnqLFznuZU). //! //! A Complete Sample //! ----------------- //! //! ### Parameter Tuning //! //! Surface matching module treats its parameters relative to the model diameter (diameter of the axis //! parallel bounding box), whenever it can. This makes the parameters independent from the model size. //! This is why, both model and scene cloud were subsampled such that all points have a minimum distance //! of , where  is the distance along a given //! dimension. All three dimensions are sampled in similar manner. For example, if //!  is set to 0.05 and the diameter of model is 1m (1000mm), the points sampled //! from the object's surface will be approximately 50 mm apart. From another point of view, if the //! sampling RelativeSamplingStep is set to 0.05, at most  model points are generated //! (depending on how the model fills in the volume). Consequently this results in at most 8000x8000 //! pairs. In practice, because the models are not uniformly distributed over a rectangular prism, much //! less points are to be expected. Decreasing this value, results in more model points and thus a more //! accurate representation. However, note that number of point pair features to be computed is now //! quadratically increased as the complexity is O(N\^2). This is especially a concern for 32 bit //! systems, where large models can easily overshoot the available memory. Typically, values in the //! range of 0.025 - 0.05 seem adequate for most of the applications, where the default value is 0.03. //! (Note that there is a difference in this paremeter with the one presented in [drost2010](https://docs.opencv.org/4.3.0/d0/de3/citelist.html#CITEREF_drost2010) . In //! [drost2010](https://docs.opencv.org/4.3.0/d0/de3/citelist.html#CITEREF_drost2010) a uniform cuboid is used for quantization and model diameter is used for reference of //! sampling. In my implementation, the cuboid is a rectangular prism, and each dimension is quantized //! independently. I do not take reference from the diameter but along the individual dimensions. //! //! It would very wise to remove the outliers from the model and prepare an ideal model initially. This //! is because, the outliers directly affect the relative computations and degrade the matching //! accuracy. //! //! During runtime stage, the scene is again sampled by , as described above. //! However this time, only a portion of the scene points are used as reference. This portion is //! controlled by the parameter , where //! . In other words, if the //! , the subsampled scene will once again be uniformly sampled to //! 1/5 of the number of points. Maximum value of this parameter is 1 and increasing this parameter also //! increases the stability, but decreases the speed. Again, because of the initial scene-independent //! relative sampling, fine tuning this parameter is not a big concern. This would only be an issue when //! the model shape occupies a volume uniformly, or when the model shape is condensed in a tiny place //! within the quantization volume (e.g. The octree representation would have too much empty cells). //! //!  acts as a step of discretization over the hash table. The point pair features //! are quantized to be mapped to the buckets of the hashtable. This discretization involves a //! multiplication and a casting to the integer. Adjusting RelativeDistanceStep in theory controls the //! collision rate. Note that, more collisions on the hashtable results in less accurate estimations. //! Reducing this parameter increases the affect of quantization but starts to assign non-similar point //! pairs to the same bins. Increasing it however, wanes the ability to group the similar pairs. //! Generally, because during the sampling stage, the training model points are selected uniformly with //! a distance controlled by RelativeSamplingStep, RelativeDistanceStep is expected to equate to this //! value. Yet again, values in the range of 0.025-0.05 are sensible. This time however, when the model //! is dense, it is not advised to decrease this value. For noisy scenes, the value can be increased to //! improve the robustness of the matching against noisy points. use crate::{mod_prelude::*, core, sys, types}; pub mod prelude { pub use { super::Pose3DTrait, super::PoseCluster3DTrait, super::PPF3DDetectorTrait, super::ICPTrait }; } pub const ICP_ICP_SAMPLING_TYPE_GELFAND: i32 = 1; pub const ICP_ICP_SAMPLING_TYPE_UNIFORM: i32 = 0; pub type key_type = u32; pub type Pose3DPtr = core::Ptr::<crate::surface_matching::Pose3D>; pub type PoseCluster3DPtr = core::Ptr::<crate::surface_matching::PoseCluster3D>; /// This class implements a very efficient and robust variant of the iterative closest point (ICP) algorithm. /// The task is to register a 3D model (or point cloud) against a set of noisy target data. The variants are put together /// by myself after certain tests. The task is to be able to match partial, noisy point clouds in cluttered scenes, quickly. /// You will find that my emphasis is on the performance, while retaining the accuracy. /// This implementation is based on Tolga Birdal's MATLAB implementation in here: /// http://www.mathworks.com/matlabcentral/fileexchange/47152-icp-registration-using-efficient-variants-and-multi-resolution-scheme /// The main contributions come from: /// 1. Picky ICP: /// http://www5.informatik.uni-erlangen.de/Forschung/Publikationen/2003/Zinsser03-ARI.pdf /// 2. Efficient variants of the ICP Algorithm: /// http://docs.happycoders.org/orgadoc/graphics/imaging/fasticp_paper.pdf /// 3. Geometrically Stable Sampling for the ICP Algorithm: https://graphics.stanford.edu/papers/stabicp/stabicp.pdf /// 4. Multi-resolution registration: /// http://www.cvl.iis.u-tokyo.ac.jp/~oishi/Papers/Alignment/Jost_MultiResolutionICP_3DIM03.pdf /// 5. Linearization of Point-to-Plane metric by Kok Lim Low: /// https://www.comp.nus.edu.sg/~lowkl/publications/lowk_point-to-plane_icp_techrep.pdf pub trait ICPTrait { fn as_raw_ICP(&self) -> *const c_void; fn as_raw_mut_ICP(&mut self) -> *mut c_void; /// \brief Perform registration /// /// ## Parameters /// * srcPC: The input point cloud for the model. Expected to have the normals (Nx6). Currently, /// CV_32F is the only supported data type. /// * dstPC: The input point cloud for the scene. It is assumed that the model is registered on the scene. Scene remains static. Expected to have the normals (Nx6). Currently, CV_32F is the only supported data type. /// * residual:[out] The output registration error. /// * pose:[out] Transformation between srcPC and dstPC. /// \return On successful termination, the function returns 0. /// /// \details It is assumed that the model is registered on the scene. Scene remains static, while the model transforms. The output poses transform the models onto the scene. Because of the point to plane minimization, the scene is expected to have the normals available. Expected to have the normals (Nx6). fn register_model_to_scene(&mut self, src_pc: &core::Mat, dst_pc: &core::Mat, residual: &mut f64, pose: &mut core::Matx44d) -> Result<i32> { unsafe { sys::cv_ppf_match_3d_ICP_registerModelToScene_const_MatR_const_MatR_doubleR_Matx44dR(self.as_raw_mut_ICP(), src_pc.as_raw_Mat(), dst_pc.as_raw_Mat(), residual, pose) }.into_result() } /// \brief Perform registration with multiple initial poses /// /// ## Parameters /// * srcPC: The input point cloud for the model. Expected to have the normals (Nx6). Currently, /// CV_32F is the only supported data type. /// * dstPC: The input point cloud for the scene. Currently, CV_32F is the only supported data type. /// @param [in,out] poses Input poses to start with but also list output of poses. /// \return On successful termination, the function returns 0. /// /// \details It is assumed that the model is registered on the scene. Scene remains static, while the model transforms. The output poses transform the models onto the scene. Because of the point to plane minimization, the scene is expected to have the normals available. Expected to have the normals (Nx6). fn register_model_to_scene_vec(&mut self, src_pc: &core::Mat, dst_pc: &core::Mat, poses: &mut core::Vector::<crate::surface_matching::Pose3DPtr>) -> Result<i32> { unsafe { sys::cv_ppf_match_3d_ICP_registerModelToScene_const_MatR_const_MatR_vector_Pose3DPtr_R(self.as_raw_mut_ICP(), src_pc.as_raw_Mat(), dst_pc.as_raw_Mat(), poses.as_raw_mut_VectorOfPose3DPtr()) }.into_result() } } /// This class implements a very efficient and robust variant of the iterative closest point (ICP) algorithm. /// The task is to register a 3D model (or point cloud) against a set of noisy target data. The variants are put together /// by myself after certain tests. The task is to be able to match partial, noisy point clouds in cluttered scenes, quickly. /// You will find that my emphasis is on the performance, while retaining the accuracy. /// This implementation is based on Tolga Birdal's MATLAB implementation in here: /// http://www.mathworks.com/matlabcentral/fileexchange/47152-icp-registration-using-efficient-variants-and-multi-resolution-scheme /// The main contributions come from: /// 1. Picky ICP: /// http://www5.informatik.uni-erlangen.de/Forschung/Publikationen/2003/Zinsser03-ARI.pdf /// 2. Efficient variants of the ICP Algorithm: /// http://docs.happycoders.org/orgadoc/graphics/imaging/fasticp_paper.pdf /// 3. Geometrically Stable Sampling for the ICP Algorithm: https://graphics.stanford.edu/papers/stabicp/stabicp.pdf /// 4. Multi-resolution registration: /// http://www.cvl.iis.u-tokyo.ac.jp/~oishi/Papers/Alignment/Jost_MultiResolutionICP_3DIM03.pdf /// 5. Linearization of Point-to-Plane metric by Kok Lim Low: /// https://www.comp.nus.edu.sg/~lowkl/publications/lowk_point-to-plane_icp_techrep.pdf pub struct ICP { ptr: *mut c_void } opencv_type_boxed! { ICP } impl Drop for ICP { fn drop(&mut self) { extern "C" { fn cv_ICP_delete(instance: *mut c_void); } unsafe { cv_ICP_delete(self.as_raw_mut_ICP()) }; } } impl ICP { #[inline] pub fn as_raw_ICP(&self) -> *const c_void { self.as_raw() } #[inline] pub fn as_raw_mut_ICP(&mut self) -> *mut c_void { self.as_raw_mut() } } unsafe impl Send for ICP {} impl crate::surface_matching::ICPTrait for ICP { #[inline] fn as_raw_ICP(&self) -> *const c_void { self.as_raw() } #[inline] fn as_raw_mut_ICP(&mut self) -> *mut c_void { self.as_raw_mut() } } impl ICP { pub fn default() -> Result<crate::surface_matching::ICP> { unsafe { sys::cv_ppf_match_3d_ICP_ICP() }.into_result().map(|r| unsafe { crate::surface_matching::ICP::opencv_from_extern(r) } ) } /// \brief ICP constructor with default arguments. /// ## Parameters /// * iterations: /// * tolerence: Controls the accuracy of registration at each iteration of ICP. /// * rejectionScale: Robust outlier rejection is applied for robustness. This value /// actually corresponds to the standard deviation coefficient. Points with /// rejectionScale * &sigma are ignored during registration. /// * numLevels: Number of pyramid levels to proceed. Deep pyramids increase speed but /// decrease accuracy. Too coarse pyramids might have computational overhead on top of the /// inaccurate registrtaion. This parameter should be chosen to optimize a balance. Typical /// values range from 4 to 10. /// * sampleType: Currently this parameter is ignored and only uniform sampling is /// applied. Leave it as 0. /// * numMaxCorr: Currently this parameter is ignored and only PickyICP is applied. Leave it as 1. /// /// ## C++ default parameters /// * tolerence: 0.05f /// * rejection_scale: 2.5f /// * num_levels: 6 /// * sample_type: ICP::ICP_SAMPLING_TYPE_UNIFORM /// * num_max_corr: 1 pub fn new(iterations: i32, tolerence: f32, rejection_scale: f32, num_levels: i32, sample_type: i32, num_max_corr: i32) -> Result<crate::surface_matching::ICP> { unsafe { sys::cv_ppf_match_3d_ICP_ICP_const_int_const_float_const_float_const_int_const_int_const_int(iterations, tolerence, rejection_scale, num_levels, sample_type, num_max_corr) }.into_result().map(|r| unsafe { crate::surface_matching::ICP::opencv_from_extern(r) } ) } } /// Class, allowing the load and matching 3D models. /// Typical Use: /// ```ignore /// // Train a model /// ppf_match_3d::PPF3DDetector detector(0.05, 0.05); /// detector.trainModel(pc); /// // Search the model in a given scene /// vector<Pose3DPtr> results; /// detector.match(pcTest, results, 1.0/5.0,0.05); /// ``` /// pub trait PPF3DDetectorTrait { fn as_raw_PPF3DDetector(&self) -> *const c_void; fn as_raw_mut_PPF3DDetector(&mut self) -> *mut c_void; /// Set the parameters for the search /// ## Parameters /// * positionThreshold: Position threshold controlling the similarity of translations. Depends on the units of calibration/model. /// * rotationThreshold: Position threshold controlling the similarity of rotations. This parameter can be perceived as a threshold over the difference of angles /// * useWeightedClustering: The algorithm by default clusters the poses without weighting. A non-zero value would indicate that the pose clustering should take into account the number of votes as the weights and perform a weighted averaging instead of a simple one. /// /// ## C++ default parameters /// * position_threshold: -1 /// * rotation_threshold: -1 /// * use_weighted_clustering: false fn set_search_params(&mut self, position_threshold: f64, rotation_threshold: f64, use_weighted_clustering: bool) -> Result<()> { unsafe { sys::cv_ppf_match_3d_PPF3DDetector_setSearchParams_const_double_const_double_const_bool(self.as_raw_mut_PPF3DDetector(), position_threshold, rotation_threshold, use_weighted_clustering) }.into_result() } /// \brief Trains a new model. /// /// ## Parameters /// * Model: The input point cloud with normals (Nx6) /// /// \details Uses the parameters set in the constructor to downsample and learn a new model. When the model is learnt, the instance gets ready for calling "match". fn train_model(&mut self, model: &core::Mat) -> Result<()> { unsafe { sys::cv_ppf_match_3d_PPF3DDetector_trainModel_const_MatR(self.as_raw_mut_PPF3DDetector(), model.as_raw_Mat()) }.into_result() } /// \brief Matches a trained model across a provided scene. /// /// ## Parameters /// * scene: Point cloud for the scene /// * results:[out] List of output poses /// * relativeSceneSampleStep: The ratio of scene points to be used for the matching after sampling with relativeSceneDistance. For example, if this value is set to 1.0/5.0, every 5th point from the scene is used for pose estimation. This parameter allows an easy trade-off between speed and accuracy of the matching. Increasing the value leads to less points being used and in turn to a faster but less accurate pose computation. Decreasing the value has the inverse effect. /// * relativeSceneDistance: Set the distance threshold relative to the diameter of the model. This parameter is equivalent to relativeSamplingStep in the training stage. This parameter acts like a prior sampling with the relativeSceneSampleStep parameter. /// /// ## C++ default parameters /// * relative_scene_sample_step: 1.0/5.0 /// * relative_scene_distance: 0.03 fn match_(&mut self, scene: &core::Mat, results: &mut core::Vector::<crate::surface_matching::Pose3DPtr>, relative_scene_sample_step: f64, relative_scene_distance: f64) -> Result<()> { unsafe { sys::cv_ppf_match_3d_PPF3DDetector_match_const_MatR_vector_Pose3DPtr_R_const_double_const_double(self.as_raw_mut_PPF3DDetector(), scene.as_raw_Mat(), results.as_raw_mut_VectorOfPose3DPtr(), relative_scene_sample_step, relative_scene_distance) }.into_result() } } /// Class, allowing the load and matching 3D models. /// Typical Use: /// ```ignore /// // Train a model /// ppf_match_3d::PPF3DDetector detector(0.05, 0.05); /// detector.trainModel(pc); /// // Search the model in a given scene /// vector<Pose3DPtr> results; /// detector.match(pcTest, results, 1.0/5.0,0.05); /// ``` /// pub struct PPF3DDetector { ptr: *mut c_void } opencv_type_boxed! { PPF3DDetector } impl Drop for PPF3DDetector { fn drop(&mut self) { extern "C" { fn cv_PPF3DDetector_delete(instance: *mut c_void); } unsafe { cv_PPF3DDetector_delete(self.as_raw_mut_PPF3DDetector()) }; } } impl PPF3DDetector { #[inline] pub fn as_raw_PPF3DDetector(&self) -> *const c_void { self.as_raw() } #[inline] pub fn as_raw_mut_PPF3DDetector(&mut self) -> *mut c_void { self.as_raw_mut() } } unsafe impl Send for PPF3DDetector {} impl crate::surface_matching::PPF3DDetectorTrait for PPF3DDetector { #[inline] fn as_raw_PPF3DDetector(&self) -> *const c_void { self.as_raw() } #[inline] fn as_raw_mut_PPF3DDetector(&mut self) -> *mut c_void { self.as_raw_mut() } } impl PPF3DDetector { /// \brief Empty constructor. Sets default arguments pub fn default() -> Result<crate::surface_matching::PPF3DDetector> { unsafe { sys::cv_ppf_match_3d_PPF3DDetector_PPF3DDetector() }.into_result().map(|r| unsafe { crate::surface_matching::PPF3DDetector::opencv_from_extern(r) } ) } /// Constructor with arguments /// ## Parameters /// * relativeSamplingStep: Sampling distance relative to the object's diameter. Models are first sampled uniformly in order to improve efficiency. Decreasing this value leads to a denser model, and a more accurate pose estimation but the larger the model, the slower the training. Increasing the value leads to a less accurate pose computation but a smaller model and faster model generation and matching. Beware of the memory consumption when using small values. /// * relativeDistanceStep: The discretization distance of the point pair distance relative to the model's diameter. This value has a direct impact on the hashtable. Using small values would lead to too fine discretization, and thus ambiguity in the bins of hashtable. Too large values would lead to no discrimination over the feature vectors and different point pair features would be assigned to the same bin. This argument defaults to the value of RelativeSamplingStep. For noisy scenes, the value can be increased to improve the robustness of the matching against noisy points. /// * numAngles: Set the discretization of the point pair orientation as the number of subdivisions of the angle. This value is the equivalent of RelativeDistanceStep for the orientations. Increasing the value increases the precision of the matching but decreases the robustness against incorrect normal directions. Decreasing the value decreases the precision of the matching but increases the robustness against incorrect normal directions. For very noisy scenes where the normal directions can not be computed accurately, the value can be set to 25 or 20. /// /// ## C++ default parameters /// * relative_distance_step: 0.05 /// * num_angles: 30 pub fn new(relative_sampling_step: f64, relative_distance_step: f64, num_angles: f64) -> Result<crate::surface_matching::PPF3DDetector> { unsafe { sys::cv_ppf_match_3d_PPF3DDetector_PPF3DDetector_const_double_const_double_const_double(relative_sampling_step, relative_distance_step, num_angles) }.into_result().map(|r| unsafe { crate::surface_matching::PPF3DDetector::opencv_from_extern(r) } ) } } /// Class, allowing the storage of a pose. The data structure stores both /// the quaternions and the matrix forms. It supports IO functionality together with /// various helper methods to work with poses pub trait Pose3DTrait { fn as_raw_Pose3D(&self) -> *const c_void; fn as_raw_mut_Pose3D(&mut self) -> *mut c_void; fn alpha(&self) -> f64 { unsafe { sys::cv_ppf_match_3d_Pose3D_getPropAlpha_const(self.as_raw_Pose3D()) }.into_result().expect("Infallible function failed: alpha") } fn set_alpha(&mut self, val: f64) -> () { unsafe { sys::cv_ppf_match_3d_Pose3D_setPropAlpha_double(self.as_raw_mut_Pose3D(), val) }.into_result().expect("Infallible function failed: set_alpha") } fn residual(&self) -> f64 { unsafe { sys::cv_ppf_match_3d_Pose3D_getPropResidual_const(self.as_raw_Pose3D()) }.into_result().expect("Infallible function failed: residual") } fn set_residual(&mut self, val: f64) -> () { unsafe { sys::cv_ppf_match_3d_Pose3D_setPropResidual_double(self.as_raw_mut_Pose3D(), val) }.into_result().expect("Infallible function failed: set_residual") } fn model_index(&self) -> size_t { unsafe { sys::cv_ppf_match_3d_Pose3D_getPropModelIndex_const(self.as_raw_Pose3D()) }.into_result().expect("Infallible function failed: model_index") } fn set_model_index(&mut self, val: size_t) -> () { unsafe { sys::cv_ppf_match_3d_Pose3D_setPropModelIndex_size_t(self.as_raw_mut_Pose3D(), val) }.into_result().expect("Infallible function failed: set_model_index") } fn num_votes(&self) -> size_t { unsafe { sys::cv_ppf_match_3d_Pose3D_getPropNumVotes_const(self.as_raw_Pose3D()) }.into_result().expect("Infallible function failed: num_votes") } fn set_num_votes(&mut self, val: size_t) -> () { unsafe { sys::cv_ppf_match_3d_Pose3D_setPropNumVotes_size_t(self.as_raw_mut_Pose3D(), val) }.into_result().expect("Infallible function failed: set_num_votes") } fn pose(&self) -> core::Matx44d { unsafe { sys::cv_ppf_match_3d_Pose3D_getPropPose_const(self.as_raw_Pose3D()) }.into_result().expect("Infallible function failed: pose") } fn set_pose(&mut self, val: core::Matx44d) -> () { unsafe { sys::cv_ppf_match_3d_Pose3D_setPropPose_Matx44d(self.as_raw_mut_Pose3D(), val.opencv_as_extern()) }.into_result().expect("Infallible function failed: set_pose") } fn angle(&self) -> f64 { unsafe { sys::cv_ppf_match_3d_Pose3D_getPropAngle_const(self.as_raw_Pose3D()) }.into_result().expect("Infallible function failed: angle") } fn set_angle(&mut self, val: f64) -> () { unsafe { sys::cv_ppf_match_3d_Pose3D_setPropAngle_double(self.as_raw_mut_Pose3D(), val) }.into_result().expect("Infallible function failed: set_angle") } fn t(&self) -> core::Vec3d { unsafe { sys::cv_ppf_match_3d_Pose3D_getPropT_const(self.as_raw_Pose3D()) }.into_result().expect("Infallible function failed: t") } fn set_t(&mut self, val: core::Vec3d) -> () { unsafe { sys::cv_ppf_match_3d_Pose3D_setPropT_Vec3d(self.as_raw_mut_Pose3D(), val.opencv_as_extern()) }.into_result().expect("Infallible function failed: set_t") } fn q(&self) -> core::Vec4d { unsafe { sys::cv_ppf_match_3d_Pose3D_getPropQ_const(self.as_raw_Pose3D()) }.into_result().expect("Infallible function failed: q") } fn set_q(&mut self, val: core::Vec4d) -> () { unsafe { sys::cv_ppf_match_3d_Pose3D_setPropQ_Vec4d(self.as_raw_mut_Pose3D(), val.opencv_as_extern()) }.into_result().expect("Infallible function failed: set_q") } /// \brief Updates the pose with the new one /// \param [in] NewPose New pose to overwrite fn update_pose(&mut self, new_pose: &mut core::Matx44d) -> Result<()> { unsafe { sys::cv_ppf_match_3d_Pose3D_updatePose_Matx44dR(self.as_raw_mut_Pose3D(), new_pose) }.into_result() } /// \brief Updates the pose with the new one fn update_pose_1(&mut self, new_r: &mut core::Matx33d, new_t: &mut core::Vec3d) -> Result<()> { unsafe { sys::cv_ppf_match_3d_Pose3D_updatePose_Matx33dR_Vec3dR(self.as_raw_mut_Pose3D(), new_r, new_t) }.into_result() } /// \brief Updates the pose with the new one, but this time using quaternions to represent rotation fn update_pose_quat(&mut self, q: &mut core::Vec4d, new_t: &mut core::Vec3d) -> Result<()> { unsafe { sys::cv_ppf_match_3d_Pose3D_updatePoseQuat_Vec4dR_Vec3dR(self.as_raw_mut_Pose3D(), q, new_t) }.into_result() } /// \brief Left multiplies the existing pose in order to update the transformation /// \param [in] IncrementalPose New pose to apply fn append_pose(&mut self, incremental_pose: &mut core::Matx44d) -> Result<()> { unsafe { sys::cv_ppf_match_3d_Pose3D_appendPose_Matx44dR(self.as_raw_mut_Pose3D(), incremental_pose) }.into_result() } fn print_pose(&mut self) -> Result<()> { unsafe { sys::cv_ppf_match_3d_Pose3D_printPose(self.as_raw_mut_Pose3D()) }.into_result() } fn clone(&mut self) -> Result<core::Ptr::<crate::surface_matching::Pose3D>> { unsafe { sys::cv_ppf_match_3d_Pose3D_clone(self.as_raw_mut_Pose3D()) }.into_result().map(|r| unsafe { core::Ptr::<crate::surface_matching::Pose3D>::opencv_from_extern(r) } ) } fn write_pose(&mut self, file_name: &str) -> Result<i32> { extern_container_arg!(file_name); unsafe { sys::cv_ppf_match_3d_Pose3D_writePose_const_stringR(self.as_raw_mut_Pose3D(), file_name.opencv_as_extern()) }.into_result() } fn read_pose(&mut self, file_name: &str) -> Result<i32> { extern_container_arg!(file_name); unsafe { sys::cv_ppf_match_3d_Pose3D_readPose_const_stringR(self.as_raw_mut_Pose3D(), file_name.opencv_as_extern()) }.into_result() } } /// Class, allowing the storage of a pose. The data structure stores both /// the quaternions and the matrix forms. It supports IO functionality together with /// various helper methods to work with poses pub struct Pose3D { ptr: *mut c_void } opencv_type_boxed! { Pose3D } impl Drop for Pose3D { fn drop(&mut self) { extern "C" { fn cv_Pose3D_delete(instance: *mut c_void); } unsafe { cv_Pose3D_delete(self.as_raw_mut_Pose3D()) }; } } impl Pose3D { #[inline] pub fn as_raw_Pose3D(&self) -> *const c_void { self.as_raw() } #[inline] pub fn as_raw_mut_Pose3D(&mut self) -> *mut c_void { self.as_raw_mut() } } unsafe impl Send for Pose3D {} impl crate::surface_matching::Pose3DTrait for Pose3D { #[inline] fn as_raw_Pose3D(&self) -> *const c_void { self.as_raw() } #[inline] fn as_raw_mut_Pose3D(&mut self) -> *mut c_void { self.as_raw_mut() } } impl Pose3D { pub fn default() -> Result<crate::surface_matching::Pose3D> { unsafe { sys::cv_ppf_match_3d_Pose3D_Pose3D() }.into_result().map(|r| unsafe { crate::surface_matching::Pose3D::opencv_from_extern(r) } ) } /// ## C++ default parameters /// * model_index: 0 /// * num_votes: 0 pub fn new(alpha: f64, model_index: size_t, num_votes: size_t) -> Result<crate::surface_matching::Pose3D> { unsafe { sys::cv_ppf_match_3d_Pose3D_Pose3D_double_size_t_size_t(alpha, model_index, num_votes) }.into_result().map(|r| unsafe { crate::surface_matching::Pose3D::opencv_from_extern(r) } ) } } /// When multiple poses (see Pose3D) are grouped together (contribute to the same transformation) /// pose clusters occur. This class is a general container for such groups of poses. It is possible to store, /// load and perform IO on these poses. pub trait PoseCluster3DTrait { fn as_raw_PoseCluster3D(&self) -> *const c_void; fn as_raw_mut_PoseCluster3D(&mut self) -> *mut c_void; fn pose_list(&mut self) -> core::Vector::<crate::surface_matching::Pose3DPtr> { unsafe { sys::cv_ppf_match_3d_PoseCluster3D_getPropPoseList(self.as_raw_mut_PoseCluster3D()) }.into_result().map(|r| unsafe { core::Vector::<crate::surface_matching::Pose3DPtr>::opencv_from_extern(r) } ).expect("Infallible function failed: pose_list") } fn set_pose_list(&mut self, mut val: core::Vector::<crate::surface_matching::Pose3DPtr>) -> () { unsafe { sys::cv_ppf_match_3d_PoseCluster3D_setPropPoseList_vector_Pose3DPtr_(self.as_raw_mut_PoseCluster3D(), val.as_raw_mut_VectorOfPose3DPtr()) }.into_result().expect("Infallible function failed: set_pose_list") } fn num_votes(&self) -> size_t { unsafe { sys::cv_ppf_match_3d_PoseCluster3D_getPropNumVotes_const(self.as_raw_PoseCluster3D()) }.into_result().expect("Infallible function failed: num_votes") } fn set_num_votes(&mut self, val: size_t) -> () { unsafe { sys::cv_ppf_match_3d_PoseCluster3D_setPropNumVotes_size_t(self.as_raw_mut_PoseCluster3D(), val) }.into_result().expect("Infallible function failed: set_num_votes") } fn id(&self) -> i32 { unsafe { sys::cv_ppf_match_3d_PoseCluster3D_getPropId_const(self.as_raw_PoseCluster3D()) }.into_result().expect("Infallible function failed: id") } fn set_id(&mut self, val: i32) -> () { unsafe { sys::cv_ppf_match_3d_PoseCluster3D_setPropId_int(self.as_raw_mut_PoseCluster3D(), val) }.into_result().expect("Infallible function failed: set_id") } /// \brief Adds a new pose to the cluster. The pose should be "close" to the mean poses /// in order to preserve the consistency /// \param [in] newPose Pose to add to the cluster fn add_pose(&mut self, mut new_pose: crate::surface_matching::Pose3DPtr) -> Result<()> { unsafe { sys::cv_ppf_match_3d_PoseCluster3D_addPose_Pose3DPtr(self.as_raw_mut_PoseCluster3D(), new_pose.as_raw_mut_PtrOfPose3D()) }.into_result() } fn write_pose_cluster(&mut self, file_name: &str) -> Result<i32> { extern_container_arg!(file_name); unsafe { sys::cv_ppf_match_3d_PoseCluster3D_writePoseCluster_const_stringR(self.as_raw_mut_PoseCluster3D(), file_name.opencv_as_extern()) }.into_result() } fn read_pose_cluster(&mut self, file_name: &str) -> Result<i32> { extern_container_arg!(file_name); unsafe { sys::cv_ppf_match_3d_PoseCluster3D_readPoseCluster_const_stringR(self.as_raw_mut_PoseCluster3D(), file_name.opencv_as_extern()) }.into_result() } } /// When multiple poses (see Pose3D) are grouped together (contribute to the same transformation) /// pose clusters occur. This class is a general container for such groups of poses. It is possible to store, /// load and perform IO on these poses. pub struct PoseCluster3D { ptr: *mut c_void } opencv_type_boxed! { PoseCluster3D } impl Drop for PoseCluster3D { fn drop(&mut self) { extern "C" { fn cv_PoseCluster3D_delete(instance: *mut c_void); } unsafe { cv_PoseCluster3D_delete(self.as_raw_mut_PoseCluster3D()) }; } } impl PoseCluster3D { #[inline] pub fn as_raw_PoseCluster3D(&self) -> *const c_void { self.as_raw() } #[inline] pub fn as_raw_mut_PoseCluster3D(&mut self) -> *mut c_void { self.as_raw_mut() } } unsafe impl Send for PoseCluster3D {} impl crate::surface_matching::PoseCluster3DTrait for PoseCluster3D { #[inline] fn as_raw_PoseCluster3D(&self) -> *const c_void { self.as_raw() } #[inline] fn as_raw_mut_PoseCluster3D(&mut self) -> *mut c_void { self.as_raw_mut() } } impl PoseCluster3D { pub fn default() -> Result<crate::surface_matching::PoseCluster3D> { unsafe { sys::cv_ppf_match_3d_PoseCluster3D_PoseCluster3D() }.into_result().map(|r| unsafe { crate::surface_matching::PoseCluster3D::opencv_from_extern(r) } ) } pub fn new(mut new_pose: crate::surface_matching::Pose3DPtr) -> Result<crate::surface_matching::PoseCluster3D> { unsafe { sys::cv_ppf_match_3d_PoseCluster3D_PoseCluster3D_Pose3DPtr(new_pose.as_raw_mut_PtrOfPose3D()) }.into_result().map(|r| unsafe { crate::surface_matching::PoseCluster3D::opencv_from_extern(r) } ) } pub fn new_1(mut new_pose: crate::surface_matching::Pose3DPtr, new_id: i32) -> Result<crate::surface_matching::PoseCluster3D> { unsafe { sys::cv_ppf_match_3d_PoseCluster3D_PoseCluster3D_Pose3DPtr_int(new_pose.as_raw_mut_PtrOfPose3D(), new_id) }.into_result().map(|r| unsafe { crate::surface_matching::PoseCluster3D::opencv_from_extern(r) } ) } }